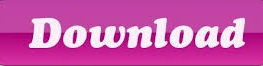
- #.SPF SEQUENCHER MEGA7 UPGRADE#
- #.SPF SEQUENCHER MEGA7 FULL#
- #.SPF SEQUENCHER MEGA7 SOFTWARE#
- #.SPF SEQUENCHER MEGA7 CODE#
Because of the extensive system memory requirements, we automatically choose a pixel format that maximizes the number of sequences displayed. When a DDB is not possible to generate, then Tree Explorer renders the tree as a device independent bitmap. For example, in a computer equipped with GeForce GT 640 graphics card, Tree Explorer successfully rendered trees with more than 100,000 sequences and responded quickly to the user scrolling and display changes. If successful, the tree image is stored in video memory, which enhances performance. To display a tree, we first evaluate if the tree can be rendered as a device-dependent bitmap (DDB), which depends on the power of the available graphics processing unit. This is made possible by our new adaptive approach to render the tree to ensure the best display quality and exploration performance. This was accomplished by replacing the native Windows scroll box with a custom virtual scroll box, which increased the number of taxa that can be displayed in the Tree Explorer window from ∼4,000 in M ega6 to greater than 100,000 sequences in M ega7.
#.SPF SEQUENCHER MEGA7 UPGRADE#
The ability to construct a phylogenetic tree of >10,000 sequences required a major upgrade of the Tree Explorer as well, because it needed to display very large trees. Therefore, M ega 7 is a significant upgrade that does not incur any discernible computational or resource penalty. However, the 32-bit M ega 6 could only carry out ML analysis for fewer than 3,000 sequences of the same length.

This is primarily because both M ega 6 and M ega 7 use 8-byte floating point data types. In addition, we compared the memory and time needs for 32- and 64-bit versions (M ega6 and M ega7, respectively), and found no significant difference for NJ and ML analyses. Computational time and peak memory showed a linear trend. We also benchmarked M ega7 for datasets with increasing number of sites.
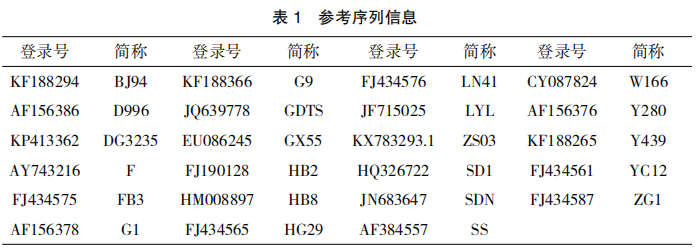
ML required an order of magnitude greater time and memory. 1 A and C ) showed a polynomial trend for NJ and a linear trend for ML. The time to complete the computation ( fig. For the Maximum Likelihood (ML) analyses, memory usage increased linearly and the peak memory usage was at 18.6 GB ( fig.
#.SPF SEQUENCHER MEGA7 FULL#
The peak memory usage was 1.7 GB for the full dataset of 10,000 rRNA sequences ( fig. For the Neighbor-Joining (NJ) method ( Saitou and Nei 1987 ), memory usage increased at a polynomial rate as the number of sequences was increased. Figure 1 shows that their computational analysis requires large amounts of memory and computing power. 2014 ) with thousands of sites and increasingly greater number of sequences (as many as 10,000). We benchmarked 64-bit M ega7 performance using 16S ribosomal RNA sequence alignments obtained from the SILVA rRNA database project ( Quast et al.
#.SPF SEQUENCHER MEGA7 CODE#
M ega ’s internal data structures have been upgraded, and the refactored source code has been tested extensively using automated test harnesses. To harness this power in evolutionary analyses, we have advanced the M ega source code to fully utilize 64-bit computing resources and memory in data handling, file processing, and evolutionary analytics. It is now common to have many gigabytes of memory with a 64-bit architecture and an operating system to match. Addressing the Need to Analyze Bigger DatasetsĬontemporary personal computers and workstations pack much greater computing power and system memory than ever before.
#.SPF SEQUENCHER MEGA7 SOFTWARE#
We have advanced the M ega software suite to address these needs of researchers performing comparative analyses of DNA and protein sequences of increasing larger datasets. Researchers also need to conduct high-throughput and scripted analyses on their operating system of choice, which requires that M ega be available in native cross-platform implementation. This necessitated technological advancement of the computation core and the user interface of M ega. Molecular Evolutionary Genetics Analysis (M ega ) software is now being applied to increasingly bigger datasets ( Kumar et al.
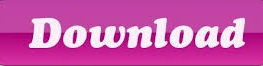